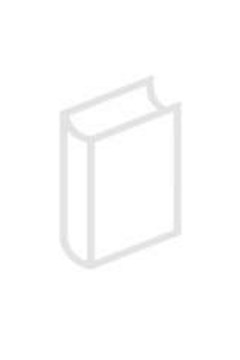
Publication details
Verlag: Springer
Ort: Berlin
Jahr: 2017
Pages: 382-393
Reihe: Lecture Notes in Computer Science
ISBN (Hardback): 9783319670072
Volle Referenz:
, "Mining semantic patterns for sentiment analysis of product reviews", in: Research and advanced technology for digital libraries, Berlin, Springer, 2017


Mining semantic patterns for sentiment analysis of product reviews
pp. 382-393
in: Jaap Kamps, Giannis Tsakonas, Yannis Manolopoulos, Lazaros Iliadis, Ioannis Karydis (eds), Research and advanced technology for digital libraries, Berlin, Springer, 2017Abstrakt
A central challenge in building sentiment classifiers using machine learning approach is the generation of discriminative features that allow sentiment to be implied. Researchers have made significant progress with various features such as n-grams, sentiment shifters, and lexicon features. However, the potential of semantics-based features in sentiment classification has not been fully explored. By integrating PropBank-based semantic parsing and class association rule (CAR) mining, this study aims to mine patterns of semantic labels from domain corpus for sentence-level sentiment analysis of product reviews. With the features generated from the semantic patterns, the F-score of the sentiment classifier was boosted to 82.31% at minimum confidence level of 0.75, which not only indicated a statistically significant improvement over the baseline classifier with unigram and negation features (F-score = 73.93%) but also surpassed the best performance obtained with other classifiers trained on generic lexicon features (F-score = 76.25%) and domain-specific lexicon features (F-score = 78.91%).
Publication details
Verlag: Springer
Ort: Berlin
Jahr: 2017
Pages: 382-393
Reihe: Lecture Notes in Computer Science
ISBN (Hardback): 9783319670072
Volle Referenz:
, "Mining semantic patterns for sentiment analysis of product reviews", in: Research and advanced technology for digital libraries, Berlin, Springer, 2017